Automating workflows for processing and classification of drone imagery to monitor grassland conservation easements
The University of Idaho’s Drone Lab has worked with the Montana Chapter of The Nature Conservancy to develop and refine workflows for automated processing of drone imagery into indicators of ecosystem health.
The Montana Chapter of The Nature Conservancy (MT-TNC) has made substantial investments in unpiloted aerial vehicle (UAV, i.e., drone) technology as a core part of its grassland monitoring programs. The University of Idaho’s Drone Lab has worked with MT-TNC to develop and refine workflows for automated processing of drone imagery to classify land cover extents and compositions. Advances in deep leaning models has provided the opportunity to explore the uses of convolutional neural networks (CNNs) to aid in vegetation monitoring and image classification.
While the adoption of image-based monitoring techniques can greatly decrease the time and effort required to collect data in the field, it increases the time and workload for extracting monitoring indicators from the images in the office. Additionally, inconsistencies in how image products are created or analyzed could result in spurious differences in the monitoring indicators. Accordingly, for image-based monitoring techniques to be widely used in monitoring programs, consistent, repeatable, and easy-to-use methods for processing the data need to be developed. This project aims to create an automated workflow to standardize the image handling process and a deep learning model for vegetation classification.monitoring needs, but that are also transferrable to other locations, ecosystems, and monitoring situations.
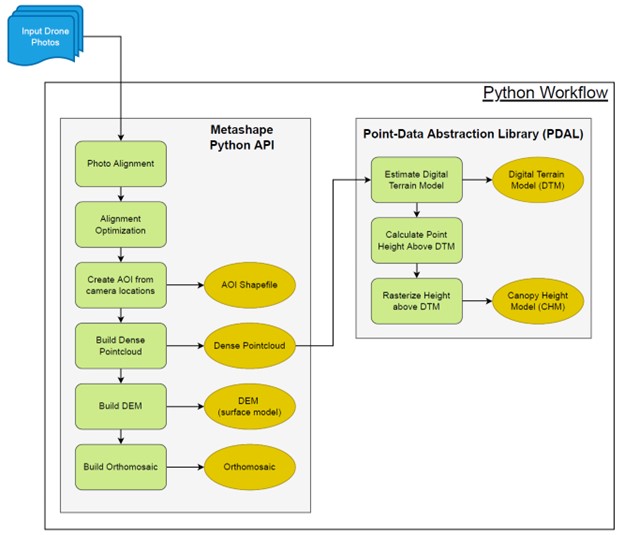
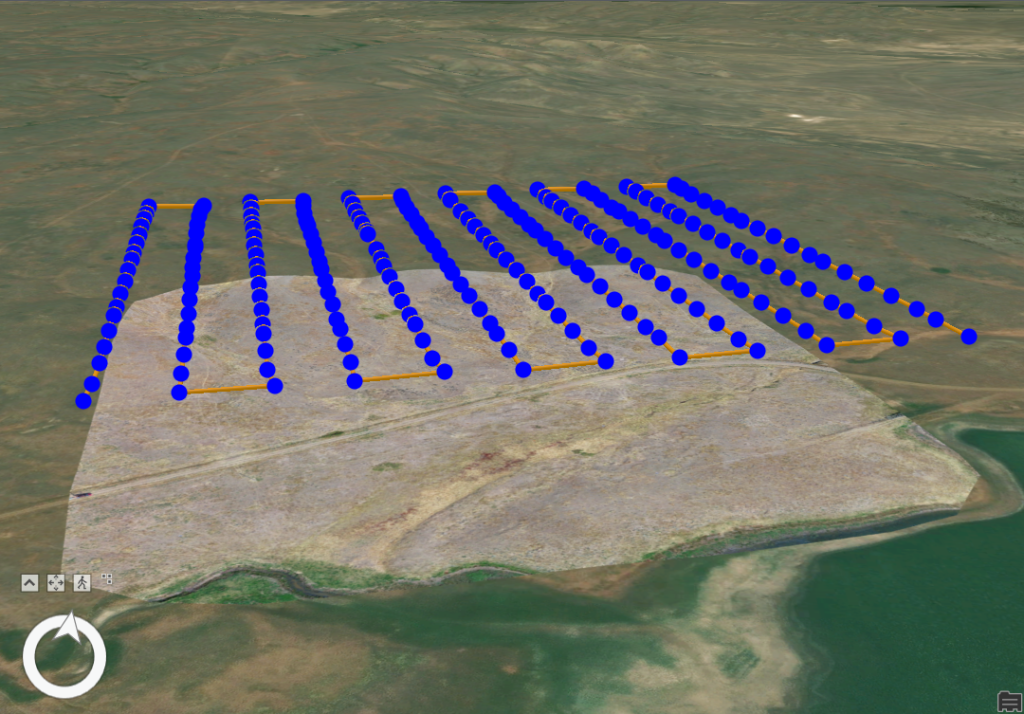